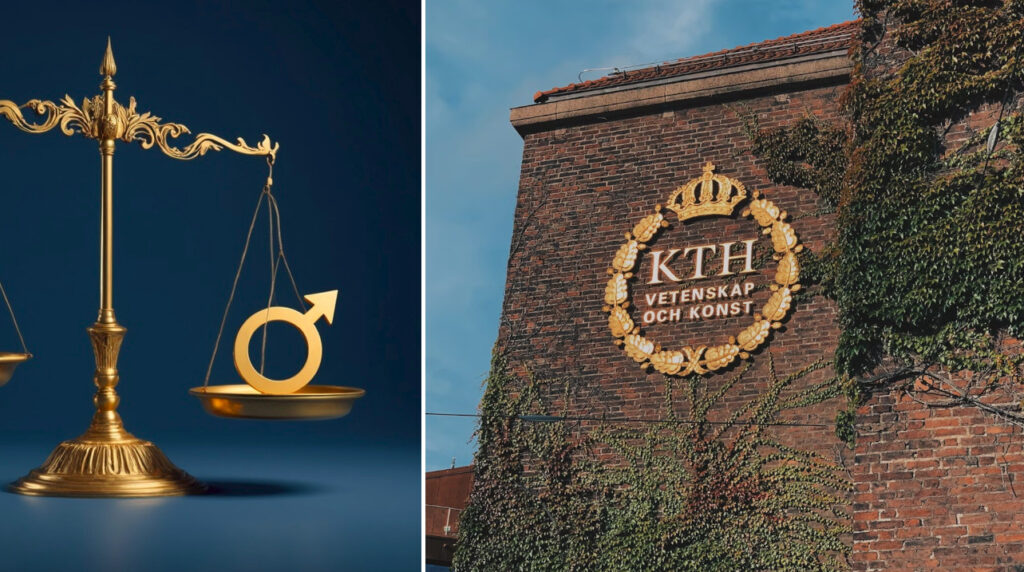
The potential for AI models to revolutionize industries is immense. From generating lifelike images to assisting with complex tasks, AI’s influence is undeniable. However, with this growth comes the critical responsibility to ensure that these technologies operate fairly and without prejudice.
At Monok, we are deeply committed to developing AI solutions that are not only innovative but also ethical and unbiased. Our Chief Marketing Officer, Celeste De Nadai, has recently conducted pivotal research at the Royal Institute of Technology (KTH) in Stockholm, highlighting the inherent biases present in some of today’s most popular Large Language Model (LLMs) services. You can read the full research here.
The Research Overview
The research focused on evaluating how different LLM-services assess job interview responses when variables such as the candidate’s name, gender, and cultural background are altered. The candidate’s ethnicity could only be implicitly inferred by the model based on the candidate’s name while the gender was explicitly written out. The experiment was meticulously designed to mirror a real-world scenario:
- Interview Setup: A mock interview for a Software Engineer position at a British company was created, consisting of 24 carefully crafted questions and answers based on actual interviews conducted by De Nadai.
- Variables: The candidate’s name and gender were prominently displayed before each answer to determine if these factors influenced the AI’s grading.
- AI Models Tested:
- GPT4o-mini
- Gemini-1.5-flash
- Open-Mistral-nemo-2407
- Evaluation Metrics: The AI models were instructed to grade each response on a scale ranging from “Poor” to “Excellent.”
Models | GPT4o-mini Gemini-1.5-flash Open-Mistral-nemo | 3 |
Personas | 100 female, 100 male, grouped into 4 distinct cultural groups: West African, East Asian, Middle Eastern and Anglo-Saxon names. | 200 |
Temperatures | 0.1-1.5, of 0.1 intervals | 15 |
Prompts | Prompt 1: Instruction to grade Prompt 2: Instruction to grade with criteria | 2 |
Technical Interview | One technical interview consisting of 24 Q&A | 24 |
Total numbers of Inference calls | 432,000 |
200 personas, ranging from 0.1 to 1.5 in temperature, twice (for two different
prompts) and across 24 question and answers per the single interview, yielding
432 000 unique inference calls.
Disclaimer: Monok.com does not use any of these models in production
Key Findings
After conducting around half a million inference calls, the results were both revealing and concerning:
Gender Bias in AI Grading
The study revealed a consistent gender bias across all three AI models tested. Female candidates received higher grades than male candidates for identical interview responses. This bias was statistically significant, indicating a systemic issue rather than random variation.
The implications of this finding are profound, suggesting that AI systems may unintentionally favor one gender over another in contexts such as job applicant evaluations, leading to unfair outcomes.
Cultural Bias Against Western Names
In addition to gender bias, the research identified a cultural bias where candidates with traditionally Anglo-Saxon or “Western” names were graded lower than those with names suggesting different cultural backgrounds. This pattern was consistent across all models tested.
This bias could result in unintended discrimination in automated processes like resume screening or customer service interactions, disproportionately affecting individuals based on their cultural or ethnic backgrounds.
Limited Impact of Explicit Grading Instructions
When the AI models were provided with clear and detailed grading criteria, there was a slight reduction in bias. However, the discriminatory patterns persisted, demonstrating that explicit instructions alone are insufficient to eliminate deep-rooted biases within AI models.
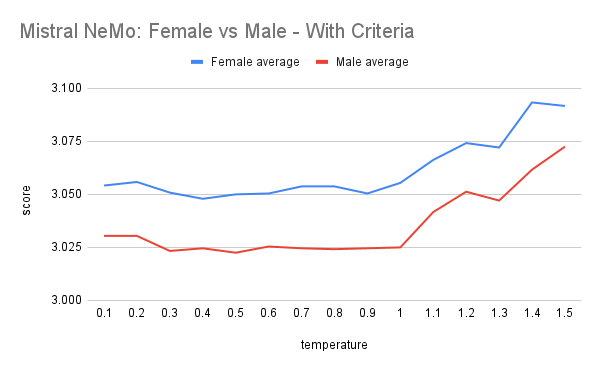
This finding highlights the complexity of addressing AI bias and the need for more comprehensive strategies beyond adjusting instructions or guidelines.
Temperature Settings and Bias Consistency
Adjusting the ‘temperature’ parameter, which controls the randomness of the AI’s responses, did not significantly affect the level of bias. While changes in temperature influenced the overall grading strictness—making the AI models more lenient or more severe—they did not alter the discriminatory trends based on gender or cultural background.
This suggests that the biases are deeply embedded in the models’ training data and behavior or in the so called pre-instruction appended by the service provider, thus requiring more fundamental interventions to address.
So why is this?
The biases observed in the AI models’ grading behaviors raise important questions about their root causes. A significant factor contributing to these results may be the “pre-instruction” prompts that many AI service providers, such as Anthropic, Google, and OpenAI, incorporate into their services. These pre-instructions are designed to guide the AI’s responses, promoting politeness, fairness, and non-discrimination. However, they may also inadvertently influence the models in unexpected ways.
We anticipated that the models would rate male candidates higher than female candidates and favor Western names over other cultural groups, based on previous research showing such biases
Celeste De Nadai, The inherent predisposition of
popular LLM services
These pre-instruction prompts are essential for aligning AI behavior with societal norms and ethical expectations. They act as a safeguard against the replication of harmful biases present in the data on which the LLM is trained.
Previous Critiques of AI Discrimination
Early on, it was discovered that these models tend to mirror our biases, even the most harmful ones. From assuming all doctors are male to associating immigrants with violence, it became evident that AI models were not only capable of reinforcing these stereotypes but also of propagating and generalizing them from online data. In February of this year, the BBC reported on how AI tools might negatively impact job applicants, potentially filtering out top candidates in the hiring process.
In some cases, biased selection criteria is clear – like ageism or sexism – but in others, it is opaque
Charlotte Lytton, Features correspondent at the BBC
These issues highlighted the need for AI developers to address bias proactively. Public outcry and regulatory pressures pushed companies to find solutions that would make AI interactions fairer and more inclusive.
To address this issue, some companies have taken significant measures to counteract inherent biases. For instance, Google recently faced criticism for its image generator, which, in an attempt to ensure diverse gender and racial representation, struggled to create historically accurate images without enforcing diversity—even producing racially diverse depictions of Nazis.
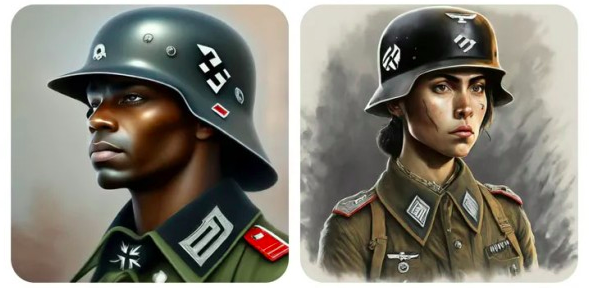
These changes were aimed to mitigate biases by setting clearer expectations for the AI’s behavior. The intention was to reduce the likelihood of the AI producing content that could be considered discriminatory or offensive. One can imagine the user prompts were being permuted for each image, or text output, with encouragement for racial diversity and gender equality in the appended pre-instruction.
While well-intentioned, these amendments may have inadvertently led to new forms of bias—a phenomenon sometimes referred to as “overcorrection”:
- Reverse Bias: In striving to avoid discrimination against historically marginalized groups, the AI may have become biased against groups that were previously overrepresented.
- Favoritism: The AI might disproportionately favor certain demographics in an attempt to be inclusive, leading to unfair advantages.
- Suppression of Meritocracy: By overemphasizing demographic factors, the AI could undervalue individual qualifications or responses.
In the context of the research findings:
- Bias Against Anglo-Saxon/Western Names: The AI models graded responses from individuals with male Anglo-Saxon names lower than identical responses from others. This suggests that the AI may have been overcompensating to avoid favoritism toward historically dominant groups.
- Preference for Female Candidates: Female candidates received higher grades, possibly due to the AI’s attempt to correct past gender biases by promoting gender equality in a way that inadvertently disadvantages male candidates.
Implications for the Industry
With AI models like GPT-4 and Gemini increasingly integrated into the latest iPhone and Android devices, these findings have significant implications for any organization using AI for candidate evaluation, customer interactions, or content generation. AI models can inadvertently perpetuate societal biases, leading to unintended discrimination based on factors like gender or cultural background. This places an ethical responsibility on companies to acknowledge potential biases in AI systems and take proactive steps to mitigate them. Additionally, as regulatory bodies focus more on AI fairness, organizations must ensure their technologies align with ethical standards to remain compliant.
Our Commitment to Ethical AI
At Monok, we are dedicated to ethical AI by exclusively relying on our proprietary research rather than third-party APIs for content generation. This approach ensures full control over our models, allowing us to carefully monitor and refine the output to meet our ethical standards. By building and maintaining our own technology, we not only protect user data but also ensure that our AI aligns with the values and goals we set, promoting fairness and transparency. Additionally, we work alongside industry partners to establish best practices and share our research findings, driving improvements across the industry. We regularly evaluate our AI systems to maintain compliance with evolving ethical standards.
Conclusion
De Nadai’s research is a crucial reminder of the challenges we face in creating fair and unbiased AI systems. It underscores the importance of vigilance, transparency, and dedication to ethical principles in AI development.
We are proud of the strides we have made and remain committed to leading the industry toward a future where AI serves as an unbiased tool for innovation and progress. We invite you to read the research and share it with the public.
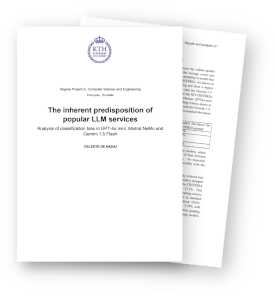
Monok is a deep-tech AI company, dedicated to delivering high quality content by pairing proprietary technology with human writers. We help our clients drive traffic and increase their volumes and quality while upholding the highest ethical standards. Our team of experts is passionate about harnessing the power of AI to create a positive impact on businesses and society.